The increasing demand for lithium-ion batteries (LIBs) to underpin the decarbonization of the transport and energy sectors is critical to expanding LIB manufacturing capacity and efficiency while concurrently reducing production costs and environmental impact.
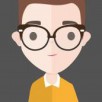
Lorem ipsum dolor sit amet, nec in adipiscing purus luctus, urna pellentesque fringilla vel, non sed arcu integer, mauris ullamcorper ante ut non torquent.
LIB manufacturing cost and CO2 emissions correlate strongly with the ‘quality’ of products and the predictability at the different production stages and handling the material waste, the employed energy, and the accumulation of failed cells. Studies indicate that up to 40% of produced LIB cells could be either defective or in need of adjustments after production. LIB production consists of various continuous and discrete process stages with different chemical, physical, electrochemical, and thermal characteristics; an optimized ‘material-to-cell’ production process is therefore not realizable without handling the numerous process interdependencies, control parameters, and uncertainties being well understood and ideally quantified.
Artificial intelligence (AI) and its high-yielding subset Machine Learning (ML) offer powerful tools to address the complex and interconnected processes associated with LIB manufacturing. Conventional LIB manufacturing data generation, analysis, and control methods are historically based on a deep qualitative understanding, where considerable time and effort are required to commission and efficiently run the production process.
AI and ML have the potential to enable researchers to conduct systematic analysis and in-situ evaluation, contributing significant benefits:
a) Efficiently handle a large amount of data. The volume of data from large-volume LIB manufacturing is growing exponentially. For example, BASF generates approximately 100 million data points per day during battery testing. AI and ML methods help to efficiently curate this data, thereby underpinning new insights into production capability, quality, and LIB performance. They help in the extraction and interpretation of information that might remain hidden with traditional data processing techniques.
b) Creation of predictive models, virtual quality gates, and digital twins. AI and especially ML algorithms when practiced on representative and rich datasets, enable the creation of models to predict LIB cell characteristics and the properties of associated intermediate products (e.g., electrode thickness, porosity, etc).
Understanding Models
While the concepts of training and validation of ML models have been well understood and transferred to the LIB manufacturing context, the explanation and interpretation of models have only recently been explored when the reliability and transparency of ML models became critical in practice.
In fact, single metrics such as prediction accuracy are not enough to describe the predictability of highly interconnected LIB manufacturing processes. The explaining ability of ML models is the capability of providing insights into the model’s behaviour, ensuring that the decisions are matched with the expectations. The explainable ML models should be able to answer ‘Why’ questions and ultimately help to build confidence within the manufacturing community.
Advances in science and technology to meet challenges Recent advances have contributed to the improvement of the design and implementation of ML models for battery manufacturing processes. The challenge of data quantity and quality in building models can be addressed via the data-mining-assisted design of experiments (DoEs), section 15. The role of ML when equipped with DoEs is considerable when exploring new materials. Unlike the traditional experimental procedures that change one control variable at a time, ML techniques can handle multiple variables that are changing at the same time.
Your Comment Please